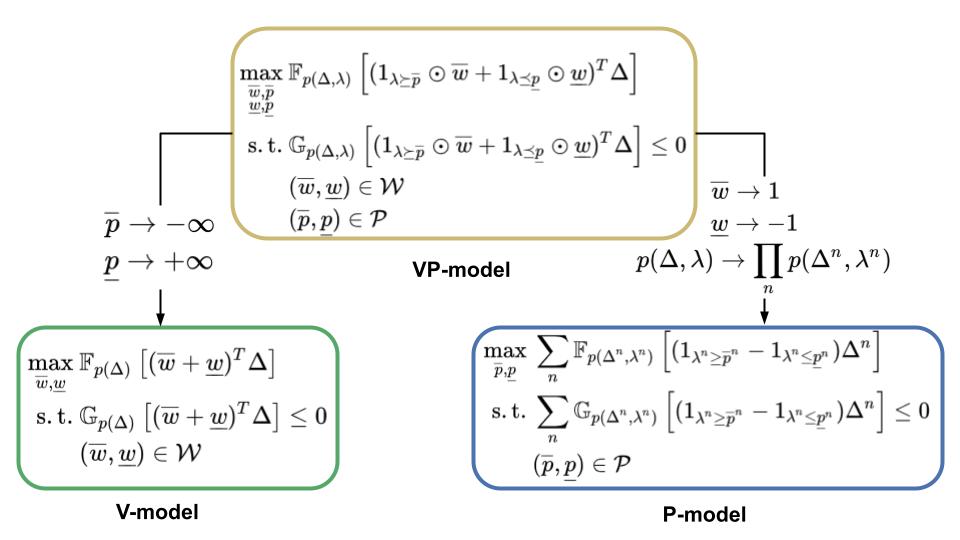
Strategic bidding
Introducing a general stochastic optimisation framework and a fast linear programming based volume-price model for convergence bidding in electric power markets.
Introducing a general stochastic optimisation framework and a fast linear programming based volume-price model for convergence bidding in electric power markets.
Predicting solutions for optimal power flow (OPF) problems by deep neural networks.
Developing and implementing efficient preconditioners for geometry optimisation and transition state search of molecular and material systems.
Introducing a potential of mean force (PMF) technique by reconstructing high dimensional free energy surfaces from instantaneous collective forces (ICF) using Gaussian process regression (GPR).
Developing machine learning based Gaussian approximation potentials (GAP) for organic molecules using high level ab initio calculations.
Introducing highly efficient collective variables for evaluating free energy profiles / surfaces of chemical reactions in complex systems.
Developing, implementing and testing hybrid quantum mechanical - molecular mechanical (QM/MM) techniques. Introducing the adaptive buffered-force QM/MM method.
Studying the origin of enzymatic catalytic effect. Using reorganization energy in rational enzyme design.
Investigating and understanding chemical reactions in enzymatic environments using molecular dynamics (MD) simulations.
Investigating and understanding solvation dynamics of electron in bulk methanol and methanol clusters.